Sleep EMA Linear Mixed Models (LMM)¶
Replication Data for Wu et al. (2020) “Multi-Modal Data Collection for Measuring Health, Behavior, and Living Environment of Large-Scale Participant Cohorts: Conceptual Framework and Findings from Deployments”: Ecological Momentary Assessment Data (Beiwe)
[1]:
import pandas as pd
import numpy as np
from glob import glob
import seaborn as sns
import matplotlib.pyplot as plt
from pymer4 import Lmer
from helpers import diagnostic_plots
from jmspack.utils import JmsColors
[2]:
if "jms_style_sheet" in plt.style.available:
plt.style.use("jms_style_sheet")
[3]:
df = (pd.read_csv("data/UT1000_ema_wide.csv")
.dropna()
.assign(**{"date": lambda d: pd.to_datetime(d["survey.date"])})
# .pipe(lambda d: d[d["survey.date"].str.contains("2019")])
.drop("survey.date", axis=1)
.pipe(lambda d: d[d["date"] <= pd.to_datetime("03-15-2019")])
.pipe(lambda d: d[d["date"] >= pd.to_datetime("02-14-2019")])
.sort_values(["pid", "date"])
.reset_index(drop=True)
)
display(df.head()); df.shape
pid | content | energy | lonely | refreshed | restful | sad | sleep | stress | date | |
---|---|---|---|---|---|---|---|---|---|---|
0 | 1193rv5x | 3.0 | 3.0 | 2.0 | 2.0 | 3.0 | 1.0 | 5.0 | 1.0 | 2019-02-14 |
1 | 1193rv5x | 2.0 | 3.0 | 3.0 | 1.0 | 2.0 | 2.0 | 6.0 | 1.0 | 2019-02-15 |
2 | 1193rv5x | 1.0 | 2.0 | 3.0 | 1.0 | 2.0 | 2.0 | 9.0 | 1.0 | 2019-02-16 |
3 | 1193rv5x | 1.0 | 1.0 | 3.0 | 2.0 | 2.0 | 3.0 | 9.0 | 2.0 | 2019-02-17 |
4 | 1193rv5x | 3.0 | 3.0 | 1.0 | 1.0 | 2.0 | 1.0 | 5.0 | 1.0 | 2019-02-18 |
[3]:
(4913, 10)
[4]:
print(df.date.min()); print(df.date.max())
2019-02-14 00:00:00
2019-03-15 00:00:00
[5]:
df.info()
<class 'pandas.core.frame.DataFrame'>
RangeIndex: 4913 entries, 0 to 4912
Data columns (total 10 columns):
# Column Non-Null Count Dtype
--- ------ -------------- -----
0 pid 4913 non-null object
1 content 4913 non-null float64
2 energy 4913 non-null float64
3 lonely 4913 non-null float64
4 refreshed 4913 non-null float64
5 restful 4913 non-null float64
6 sad 4913 non-null float64
7 sleep 4913 non-null float64
8 stress 4913 non-null float64
9 date 4913 non-null datetime64[ns]
dtypes: datetime64[ns](1), float64(8), object(1)
memory usage: 384.0+ KB
[6]:
df.describe()
[6]:
content | energy | lonely | refreshed | restful | sad | sleep | stress | |
---|---|---|---|---|---|---|---|---|
count | 4913.000000 | 4913.000000 | 4913.000000 | 4913.000000 | 4913.000000 | 4913.000000 | 4913.000000 | 4913.000000 |
mean | 1.871565 | 2.134948 | 0.513332 | 1.618563 | 1.834724 | 0.591696 | 6.237737 | 1.175453 |
std | 0.823113 | 0.830693 | 0.731918 | 0.881945 | 0.858564 | 0.773247 | 1.687056 | 0.882876 |
min | 0.000000 | 0.000000 | 0.000000 | 0.000000 | 0.000000 | 0.000000 | 0.000000 | 0.000000 |
25% | 1.000000 | 2.000000 | 0.000000 | 1.000000 | 1.000000 | 0.000000 | 5.000000 | 1.000000 |
50% | 2.000000 | 2.000000 | 0.000000 | 2.000000 | 2.000000 | 0.000000 | 6.000000 | 1.000000 |
75% | 2.000000 | 3.000000 | 1.000000 | 2.000000 | 2.000000 | 1.000000 | 7.000000 | 2.000000 |
max | 3.000000 | 4.000000 | 3.000000 | 3.000000 | 3.000000 | 3.000000 | 12.000000 | 3.000000 |
[7]:
target="sleep"
group="pid"
checkpoint="date"
feature_list=df.select_dtypes(float).drop(target, axis=1).columns.tolist()
_ = plt.figure(figsize=(5,3))
_ = sns.countplot(y=df[target])
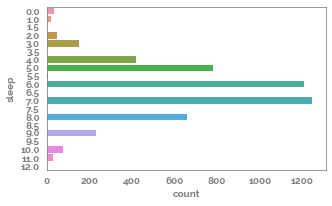
[8]:
_ = plt.figure(figsize=(20, 5))
_ = sns.stripplot(data=df.select_dtypes(float).melt(), x="variable", y="value", alpha=0.5)
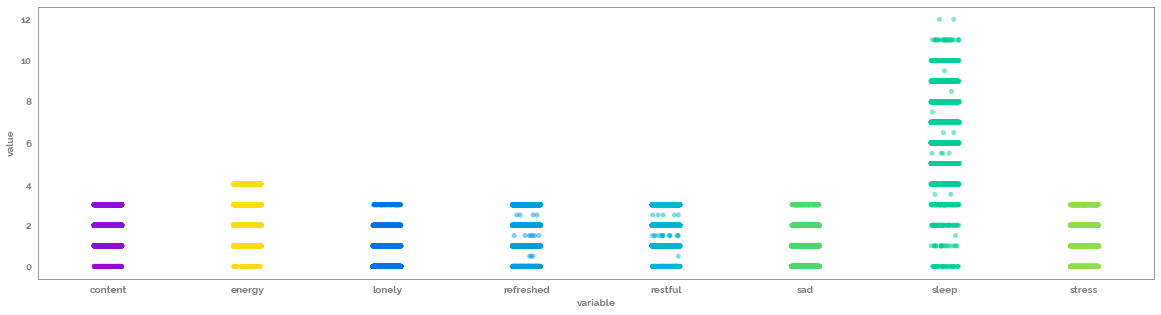
[9]:
mask = np.triu(np.ones_like(df.corr(), dtype=bool))
_ = sns.heatmap(df.corr(method="spearman"), mask=mask, annot=True)
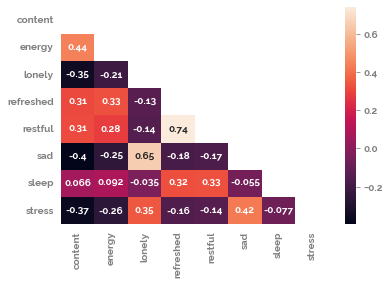
[10]:
cluster_collection = "+".join(feature_list)
cluster_collection
[10]:
'content+energy+lonely+refreshed+restful+sad+stress'
[11]:
formula_string = f"{target} ~ {cluster_collection} + (1 | {group}) + (1 | {checkpoint})"
model = Lmer(data=df, formula=formula_string)
initial_mod_fixed_eff = model.fit(no_warnings=True)
initial_mod_fixed_eff
Formula: sleep~content+energy+lonely+refreshed+restful+sad+stress+(1|pid)+(1|date)
Family: gaussian Inference: parametric
Number of observations: 4913 Groups: {'pid': 411.0, 'date': 30.0}
Log-likelihood: -8756.933 AIC: 17513.866
Random effects:
Name Var Std
pid (Intercept) 0.710 0.843
date (Intercept) 0.046 0.214
Residual 1.780 1.334
No random effect correlations specified
Fixed effects:
[11]:
Estimate | 2.5_ci | 97.5_ci | SE | DF | T-stat | P-val | Sig | |
---|---|---|---|---|---|---|---|---|
(Intercept) | 4.929 | 4.690 | 5.169 | 0.122 | 1006.443 | 40.325 | 0.000 | *** |
content | -0.064 | -0.135 | 0.007 | 0.036 | 4733.664 | -1.766 | 0.077 | . |
energy | 0.025 | -0.035 | 0.085 | 0.031 | 4876.512 | 0.826 | 0.409 | |
lonely | 0.026 | -0.059 | 0.110 | 0.043 | 4871.830 | 0.596 | 0.551 | |
refreshed | 0.338 | 0.268 | 0.408 | 0.036 | 4755.562 | 9.434 | 0.000 | *** |
restful | 0.433 | 0.361 | 0.505 | 0.037 | 4766.650 | 11.838 | 0.000 | *** |
sad | 0.010 | -0.070 | 0.090 | 0.041 | 4878.341 | 0.237 | 0.813 | |
stress | -0.040 | -0.099 | 0.019 | 0.030 | 4885.360 | -1.327 | 0.184 |
[12]:
cluster_collection="refreshed+restful"
formula_string = f"{target} ~ {cluster_collection} + (1 | {group}) + (1 | {checkpoint})"
model = Lmer(data=df, formula=formula_string)
fixed_eff = model.fit(no_warnings=True)
fixed_eff
Formula: sleep~refreshed+restful+(1|pid)+(1|date)
Family: gaussian Inference: parametric
Number of observations: 4913 Groups: {'pid': 411.0, 'date': 30.0}
Log-likelihood: -8747.223 AIC: 17494.446
Random effects:
Name Var Std
pid (Intercept) 0.714 0.845
date (Intercept) 0.046 0.214
Residual 1.780 1.334
No random effect correlations specified
Fixed effects:
[12]:
Estimate | 2.5_ci | 97.5_ci | SE | DF | T-stat | P-val | Sig | |
---|---|---|---|---|---|---|---|---|
(Intercept) | 4.840 | 4.685 | 4.996 | 0.079 | 228.722 | 61.103 | 0.0 | *** |
refreshed | 0.339 | 0.270 | 0.409 | 0.035 | 4781.391 | 9.574 | 0.0 | *** |
restful | 0.429 | 0.357 | 0.500 | 0.036 | 4786.763 | 11.765 | 0.0 | *** |
[13]:
try:
fig, axs = diagnostic_plots(model_fit=model,
X=None,
y=None,
figsize = (8,8),
limit_cooks_plot = False,
subplot_adjust_args={"wspace": 0.3, "hspace": 0.3}
)
except:
print("The function did not run - this is likely due to multiple random effects being specified")
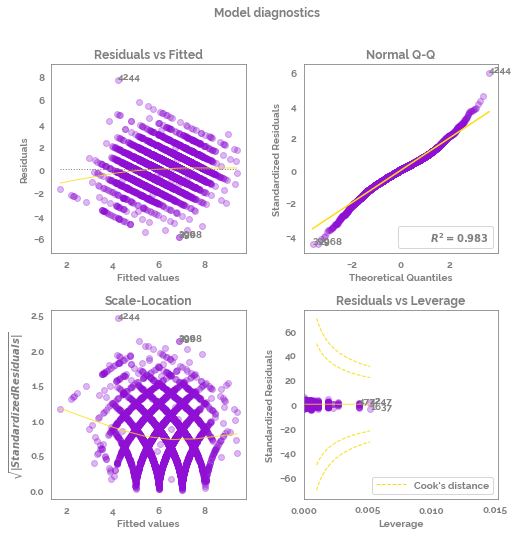
[14]:
def r2_GLMM(model):
sigma2_fe = model.design_matrix.multiply(model.fixef[0].mean()).var().sum()
sigma2_re = model.ranef_var.loc[group, "Var"]
sigma2_ol = model.ranef_var.loc["Residual", "Var"]
r2_marginal = (sigma2_fe) / (sigma2_fe + sigma2_re + sigma2_ol)
r2_conditional = (sigma2_fe + sigma2_re) / (sigma2_fe + sigma2_re + sigma2_ol)
return pd.DataFrame({"Marginal_R_squared": r2_marginal,
"Conditional_R_squared": r2_conditional},
index=[0])
Conditional and marginal R squared¶
based on Nakagawa, S., & Schielzeth, H. (2013). A general and simple method for obtaining R2 from generalized linear mixed‐effects models. Methods in ecology and evolution, 4(2), 133-142.
Marginal R-squared is the R-squared for just the fixed effects
Conditional R-squared is the R-squared for both the fixed effects and random effects
[15]:
r2_GLMM(model)
[15]:
Marginal_R_squared | Conditional_R_squared | |
---|---|---|
0 | 0.082767 | 0.34553 |
[16]:
predict_df = df.assign(**{f"{target}_fitted": model.fits})
[19]:
_ = plt.figure(figsize=(10, 4))
ax = sns.lineplot(data=predict_df,
x=checkpoint,
y=target,
hue=group,
palette=list(np.repeat(JmsColors.OFFWHITE, repeats=predict_df[group].nunique())),
legend=False,
alpha=0.4)
_ = sns.lineplot(data=predict_df,
x=checkpoint,
y=target,
color=JmsColors.DARKBLUE,
ax=ax)
_ = sns.lineplot(data=predict_df,
x=checkpoint,
y=f"{target}_fitted",
color=JmsColors.PURPLE,
ax=ax)
_ = plt.xticks(rotation=90)
_ = plt.plot([], [], label=f"{group} per user", c=JmsColors.OFFWHITE)
_ = plt.plot([], [], label=f"{group} true", c=JmsColors.DARKBLUE)
_ = plt.plot([], [], label=f"{group} fitted", c=JmsColors.PURPLE)
_ = plt.legend()
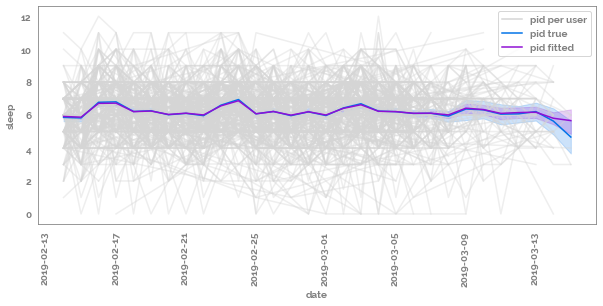
[18]:
_ = sns.lmplot(data=predict_df, x=target, y=f"{target}_fitted", hue=group, legend=False)
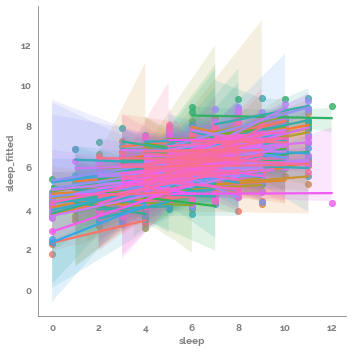