Methodology¶
The expected content of the notebook:¶
- Detection of time series changes in facial action units
Data - data frame of facial action units of James (using OpenFace for windows)¶
[1]:
import sys
import os
import warnings
import pandas as pd
from pandas.io.json import json_normalize #package for flattening json in pandas df
from ast import literal_eval
import numpy as np
import matplotlib.pyplot as plt
import seaborn as sns
from sklearn.preprocessing import MinMaxScaler
from sklearn.feature_selection import VarianceThreshold
# import CRD_functions as crd
from jmspack.NLTSA import (ts_levels,
distribution_uniformity,
fluctuation_intensity,
complexity_resonance,
complexity_resonance_diagram,
cumulative_complexity_peaks,
cumulative_complexity_peaks_plot)
from sinfo import sinfo
Display the session info of the notebook¶
[2]:
sinfo()
The `sinfo` package has changed name and is now called `session_info` to become more discoverable and self-explanatory. The `sinfo` PyPI package will be kept around to avoid breaking old installs and you can downgrade to 0.3.2 if you want to use it without seeing this message. For the latest features and bug fixes, please install `session_info` instead. The usage and defaults also changed slightly, so please review the latest README at https://gitlab.com/joelostblom/session_info.
-----
jmspack 0.0.3
matplotlib 3.3.4
numpy 1.19.2
pandas 1.2.3
seaborn 0.11.1
sinfo 0.3.4
sklearn 0.24.2
-----
IPython 7.21.0
jupyter_client 6.1.7
jupyter_core 4.7.1
jupyterlab 3.0.11
notebook 6.3.0
-----
Python 3.8.8 (default, Feb 24 2021, 15:54:32) [MSC v.1928 64 bit (AMD64)]
Windows-10-10.0.19041-SP0
16 logical CPU cores, AMD64 Family 23 Model 113 Stepping 0, AuthenticAMD
-----
Session information updated at 2021-07-11 22:59
[3]:
# ========================
# PERSONAL PREFERENCES
# Set pandas output preferences
pd.set_option('display.max_rows', 500)
pd.set_option('display.max_columns', 1000)
pd.set_option('display.width', 1000)
# Set seaborn plot style
plt.style.use('seaborn')
# ========================
[4]:
# read in data and sort data frame based on frame
jms_facs = pd.read_csv("data/FACs_Test2.csv").sort_values(by=['frame'])
[5]:
eye_cols = jms_facs.filter(regex="eye").columns.tolist()
au_cols = jms_facs.filter(regex="AU").columns.tolist()
[6]:
# select only the action units from the df
# jms_au = jms_facs[eye_cols + au_cols]
jms_au = jms_facs[au_cols]
[7]:
jms_au = jms_au[800:1400]
jms_au.head()
[7]:
AU01_r | AU02_r | AU04_r | AU05_r | AU06_r | AU07_r | AU09_r | AU10_r | AU12_r | AU14_r | AU15_r | AU17_r | AU20_r | AU23_r | AU25_r | AU26_r | AU45_r | AU01_c | AU02_c | AU04_c | AU05_c | AU06_c | AU07_c | AU09_c | AU10_c | AU12_c | AU14_c | AU15_c | AU17_c | AU20_c | AU23_c | AU25_c | AU26_c | AU28_c | AU45_c | |
---|---|---|---|---|---|---|---|---|---|---|---|---|---|---|---|---|---|---|---|---|---|---|---|---|---|---|---|---|---|---|---|---|---|---|---|
800 | 1.56 | 0.0 | 2.68 | 2.05 | 0.0 | 0.0 | 0.0 | 0.0 | 0.0 | 0.88 | 0.0 | 2.02 | 1.20 | 0.0 | 0.86 | 0.06 | 0.0 | 0.0 | 0.0 | 1.0 | 0.0 | 0.0 | 0.0 | 0.0 | 0.0 | 0.0 | 1.0 | 1.0 | 1.0 | 0.0 | 1.0 | 0.0 | 1.0 | 0.0 | 0.0 |
801 | 1.82 | 0.0 | 2.79 | 2.02 | 0.0 | 0.0 | 0.0 | 0.0 | 0.0 | 0.92 | 0.0 | 2.12 | 1.06 | 0.0 | 0.70 | 0.11 | 0.0 | 0.0 | 0.0 | 1.0 | 0.0 | 0.0 | 0.0 | 0.0 | 0.0 | 0.0 | 1.0 | 1.0 | 1.0 | 0.0 | 1.0 | 0.0 | 1.0 | 0.0 | 0.0 |
802 | 1.95 | 0.0 | 2.91 | 1.95 | 0.0 | 0.0 | 0.0 | 0.0 | 0.0 | 1.07 | 0.0 | 2.06 | 0.99 | 0.0 | 0.55 | 0.23 | 0.0 | 0.0 | 0.0 | 1.0 | 0.0 | 0.0 | 0.0 | 0.0 | 0.0 | 0.0 | 1.0 | 1.0 | 1.0 | 0.0 | 1.0 | 0.0 | 1.0 | 0.0 | 0.0 |
803 | 1.97 | 0.0 | 2.93 | 2.04 | 0.0 | 0.0 | 0.0 | 0.0 | 0.0 | 1.13 | 0.0 | 1.96 | 1.06 | 0.0 | 0.50 | 0.33 | 0.0 | 0.0 | 0.0 | 1.0 | 0.0 | 0.0 | 0.0 | 0.0 | 0.0 | 0.0 | 1.0 | 1.0 | 1.0 | 0.0 | 1.0 | 0.0 | 1.0 | 0.0 | 0.0 |
804 | 1.96 | 0.0 | 3.02 | 2.19 | 0.0 | 0.0 | 0.0 | 0.0 | 0.0 | 1.12 | 0.0 | 1.99 | 1.02 | 0.0 | 0.50 | 0.31 | 0.0 | 0.0 | 0.0 | 1.0 | 1.0 | 0.0 | 0.0 | 0.0 | 0.0 | 0.0 | 1.0 | 1.0 | 1.0 | 0.0 | 1.0 | 0.0 | 1.0 | 0.0 | 0.0 |
[8]:
jms_au.shape
[8]:
(600, 35)
[9]:
from sklearn.preprocessing import MinMaxScaler
def variance_threshold(df:pd.DataFrame,
threshold: str):
scaled_df = pd.DataFrame(MinMaxScaler()
.fit_transform(
df.select_dtypes("number")
),
columns=df.columns)
summary_df = (scaled_df
.var()
.to_frame(name="variance")
.assign(feature_type=df.dtypes)
.assign(discard=lambda x: x["variance"] < threshold)
)
return summary_df
[10]:
def nunique_threshold(df:pd.DataFrame,
threshold: str):
scaled_df = pd.DataFrame(MinMaxScaler()
.fit_transform(
df.select_dtypes("number")
),
columns=df.columns)
summary_df = (scaled_df
.apply(lambda x: x.nunique())
.to_frame(name="nunique")
.assign(feature_type=df.dtypes)
.assign(discard=lambda x: x["nunique"] < threshold)
)
return summary_df
[11]:
variance_summary = variance_threshold(df = jms_au,
threshold = 0.01)
var_drop_columns=variance_summary[variance_summary["discard"]].index.tolist()
nunique_summary = nunique_threshold(df=jms_au, threshold=3)
nunique_drop_columns=nunique_summary[nunique_summary["discard"]].index.tolist()
[12]:
drop_columns = list(set(var_drop_columns) | set(nunique_drop_columns))
[13]:
scaled_ts_df = pd.DataFrame(MinMaxScaler()
.fit_transform(
jms_au.drop(drop_columns, axis=1)
),
columns=jms_au.drop(drop_columns, axis=1).columns)
[14]:
scaled_ts_df.shape
[14]:
(600, 14)
[15]:
distribution_uniformity_df = pd.DataFrame(distribution_uniformity(scaled_ts_df,
win=7,
xmin=0,
xmax=1,
col_first=1,
col_last=scaled_ts_df.shape[1])
)
distribution_uniformity_df.columns=scaled_ts_df.columns.tolist()
[16]:
_ = complexity_resonance_diagram(distribution_uniformity_df,
plot_title='Distribution Uniformity Diagram')
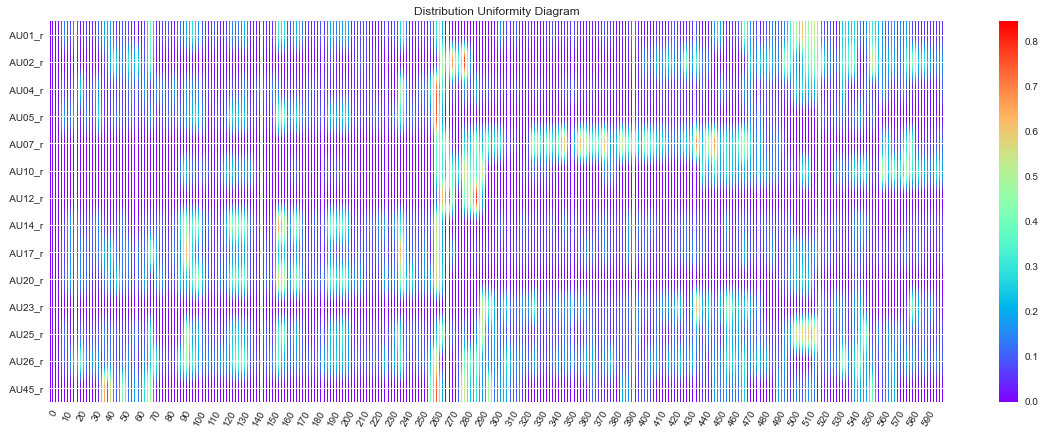
[17]:
fluctuation_intensity_df = pd.DataFrame(fluctuation_intensity(scaled_ts_df,
win=7,
xmin=0,
xmax=1,
col_first=1,
col_last=scaled_ts_df.shape[1])
)
fluctuation_intensity_df.columns=scaled_ts_df.columns.tolist()
[18]:
_ = complexity_resonance_diagram(fluctuation_intensity_df,
plot_title='Fluctuation Intensity Diagram')
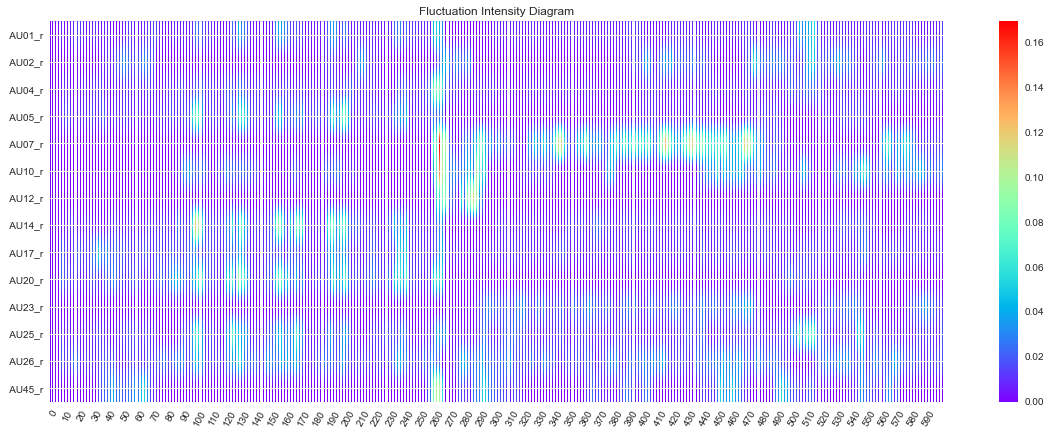
[19]:
complexity_resonance_df = complexity_resonance(distribution_uniformity_df, fluctuation_intensity_df)
[20]:
_ = complexity_resonance_diagram(complexity_resonance_df)
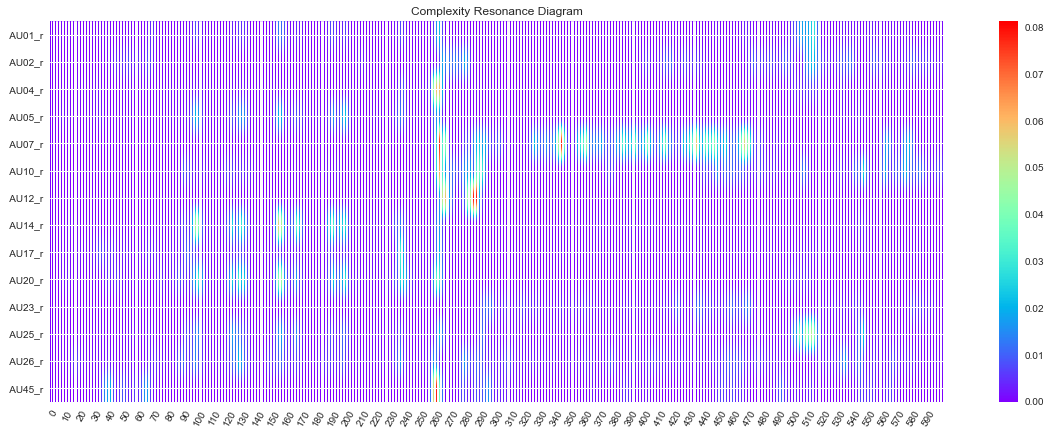
[21]:
def magnify():
return [dict(selector="th",
props=[("font-size", "4pt")]),
dict(selector="td",
props=[('padding', "0em 0em")]),
dict(selector="th:hover",
props=[("font-size", "12pt")]),
dict(selector="tr:hover td:hover",
props=[('max-width', '200px'),
('font-size', '12pt')])
]
[22]:
cmap=sns.diverging_palette(5, 250, as_cmap=True)
(complexity_resonance_df
.loc[0:100,:]
.T
.style.background_gradient(cmap, axis=1)
.set_properties(**{'max-width': '180px', 'font-size': '5pt'})
.set_caption("Complexity Resonance Df (Hover to magnify)")
.set_precision(2)
.set_table_styles(magnify())
)
[22]:
0 | 1 | 2 | 3 | 4 | 5 | 6 | 7 | 8 | 9 | 10 | 11 | 12 | 13 | 14 | 15 | 16 | 17 | 18 | 19 | 20 | 21 | 22 | 23 | 24 | 25 | 26 | 27 | 28 | 29 | 30 | 31 | 32 | 33 | 34 | 35 | 36 | 37 | 38 | 39 | 40 | 41 | 42 | 43 | 44 | 45 | 46 | 47 | 48 | 49 | 50 | 51 | 52 | 53 | 54 | 55 | 56 | 57 | 58 | 59 | 60 | 61 | 62 | 63 | 64 | 65 | 66 | 67 | 68 | 69 | 70 | 71 | 72 | 73 | 74 | 75 | 76 | 77 | 78 | 79 | 80 | 81 | 82 | 83 | 84 | 85 | 86 | 87 | 88 | 89 | 90 | 91 | 92 | 93 | 94 | 95 | 96 | 97 | 98 | 99 | 100 | |
---|---|---|---|---|---|---|---|---|---|---|---|---|---|---|---|---|---|---|---|---|---|---|---|---|---|---|---|---|---|---|---|---|---|---|---|---|---|---|---|---|---|---|---|---|---|---|---|---|---|---|---|---|---|---|---|---|---|---|---|---|---|---|---|---|---|---|---|---|---|---|---|---|---|---|---|---|---|---|---|---|---|---|---|---|---|---|---|---|---|---|---|---|---|---|---|---|---|---|---|---|---|
AU01_r | 0.00 | 0.00 | 0.00 | 0.00 | 0.00 | 0.00 | 0.00 | 0.00 | 0.00 | 0.00 | 0.00 | 0.00 | 0.00 | 0.00 | 0.00 | 0.00 | 0.00 | 0.00 | 0.00 | 0.00 | 0.00 | 0.00 | 0.00 | 0.00 | 0.00 | 0.00 | 0.00 | 0.00 | 0.00 | 0.00 | 0.00 | 0.00 | 0.00 | 0.00 | 0.00 | 0.00 | 0.00 | 0.00 | 0.00 | 0.00 | 0.00 | 0.00 | 0.00 | 0.00 | 0.00 | 0.00 | 0.00 | 0.00 | 0.00 | 0.00 | 0.00 | 0.00 | 0.00 | 0.00 | 0.00 | 0.00 | 0.00 | 0.00 | 0.00 | 0.00 | 0.00 | 0.00 | 0.00 | 0.00 | 0.00 | 0.00 | 0.00 | 0.00 | 0.01 | 0.00 | 0.00 | 0.00 | 0.00 | 0.00 | 0.00 | 0.00 | 0.00 | 0.00 | 0.00 | 0.00 | 0.00 | 0.00 | 0.00 | 0.00 | 0.00 | 0.00 | 0.00 | 0.00 | 0.00 | 0.00 | 0.00 | 0.00 | 0.00 | 0.00 | 0.00 | 0.00 | 0.00 | 0.01 | 0.00 | 0.00 | 0.00 |
AU02_r | 0.00 | 0.00 | 0.00 | 0.00 | 0.00 | 0.00 | 0.00 | 0.00 | 0.00 | 0.00 | 0.00 | 0.00 | 0.00 | 0.00 | 0.00 | 0.00 | 0.00 | 0.00 | 0.00 | 0.00 | 0.00 | 0.00 | 0.00 | 0.00 | 0.00 | 0.00 | 0.00 | 0.00 | 0.00 | 0.00 | 0.00 | 0.00 | 0.00 | 0.00 | 0.00 | 0.00 | 0.00 | 0.00 | 0.00 | 0.00 | 0.00 | 0.00 | 0.00 | 0.01 | 0.00 | 0.00 | 0.00 | 0.01 | 0.00 | 0.00 | 0.00 | 0.01 | 0.01 | 0.00 | 0.00 | 0.00 | 0.00 | 0.00 | 0.01 | 0.00 | 0.00 | 0.00 | 0.00 | 0.00 | 0.00 | 0.01 | 0.01 | 0.01 | 0.00 | 0.00 | 0.00 | 0.00 | 0.00 | 0.00 | 0.00 | 0.00 | 0.00 | 0.00 | 0.00 | 0.00 | 0.00 | 0.00 | 0.00 | 0.00 | 0.00 | 0.00 | 0.00 | 0.00 | 0.00 | 0.00 | 0.00 | 0.00 | 0.00 | 0.00 | 0.00 | 0.00 | 0.00 | 0.00 | 0.00 | 0.00 | 0.00 |
AU04_r | 0.00 | 0.00 | 0.00 | 0.00 | 0.00 | 0.00 | 0.00 | 0.00 | 0.00 | 0.00 | 0.00 | 0.00 | 0.00 | 0.00 | 0.00 | 0.00 | 0.00 | 0.00 | 0.00 | 0.00 | 0.00 | 0.00 | 0.00 | 0.00 | 0.00 | 0.00 | 0.00 | 0.00 | 0.00 | 0.00 | 0.00 | 0.00 | 0.00 | 0.00 | 0.00 | 0.00 | 0.00 | 0.00 | 0.00 | 0.00 | 0.00 | 0.00 | 0.00 | 0.00 | 0.00 | 0.00 | 0.00 | 0.00 | 0.00 | 0.00 | 0.00 | 0.00 | 0.00 | 0.00 | 0.00 | 0.00 | 0.00 | 0.00 | 0.00 | 0.00 | 0.00 | 0.00 | 0.00 | 0.00 | 0.00 | 0.00 | 0.00 | 0.00 | 0.00 | 0.00 | 0.00 | 0.00 | 0.00 | 0.00 | 0.00 | 0.00 | 0.00 | 0.00 | 0.00 | 0.00 | 0.00 | 0.00 | 0.00 | 0.00 | 0.00 | 0.00 | 0.00 | 0.00 | 0.00 | 0.00 | 0.00 | 0.00 | 0.00 | 0.00 | 0.00 | 0.00 | 0.00 | 0.00 | 0.00 | 0.00 | 0.00 |
AU05_r | 0.00 | 0.00 | 0.00 | 0.00 | 0.00 | 0.00 | 0.00 | 0.00 | 0.00 | 0.00 | 0.00 | 0.00 | 0.00 | 0.00 | 0.00 | 0.00 | 0.00 | 0.00 | 0.00 | 0.00 | 0.00 | 0.00 | 0.00 | 0.00 | 0.00 | 0.00 | 0.00 | 0.00 | 0.00 | 0.00 | 0.00 | 0.00 | 0.00 | 0.00 | 0.00 | 0.00 | 0.00 | 0.00 | 0.00 | 0.00 | 0.00 | 0.00 | 0.00 | 0.00 | 0.00 | 0.00 | 0.00 | 0.00 | 0.00 | 0.00 | 0.00 | 0.00 | 0.00 | 0.00 | 0.00 | 0.00 | 0.00 | 0.00 | 0.00 | 0.00 | 0.00 | 0.00 | 0.00 | 0.00 | 0.00 | 0.00 | 0.00 | 0.00 | 0.00 | 0.00 | 0.00 | 0.00 | 0.00 | 0.00 | 0.00 | 0.00 | 0.00 | 0.00 | 0.00 | 0.00 | 0.00 | 0.00 | 0.00 | 0.00 | 0.00 | 0.00 | 0.00 | 0.00 | 0.00 | 0.00 | 0.00 | 0.00 | 0.00 | 0.00 | 0.01 | 0.01 | 0.01 | 0.02 | 0.02 | 0.02 | 0.01 |
AU07_r | 0.00 | 0.00 | 0.00 | 0.00 | 0.00 | 0.00 | 0.00 | 0.00 | 0.00 | 0.00 | 0.00 | 0.00 | 0.00 | 0.00 | 0.00 | 0.00 | 0.00 | 0.00 | 0.00 | 0.00 | 0.00 | 0.00 | 0.00 | 0.00 | 0.00 | 0.00 | 0.00 | 0.00 | 0.00 | 0.00 | 0.00 | 0.00 | 0.00 | 0.00 | 0.00 | 0.00 | 0.00 | 0.00 | 0.00 | 0.00 | 0.00 | 0.00 | 0.00 | 0.00 | 0.00 | 0.00 | 0.00 | 0.00 | 0.00 | 0.00 | 0.00 | 0.00 | 0.00 | 0.00 | 0.00 | 0.00 | 0.00 | 0.00 | 0.00 | 0.00 | 0.00 | 0.00 | 0.00 | 0.00 | 0.00 | 0.00 | 0.00 | 0.00 | 0.00 | 0.00 | 0.00 | 0.00 | 0.00 | 0.00 | 0.00 | 0.00 | 0.00 | 0.00 | 0.00 | 0.00 | 0.00 | 0.00 | 0.00 | 0.00 | 0.00 | 0.00 | 0.00 | 0.00 | 0.00 | 0.00 | 0.00 | 0.00 | 0.00 | 0.00 | 0.00 | 0.00 | 0.00 | 0.00 | 0.00 | 0.00 | 0.00 |
AU10_r | 0.00 | 0.00 | 0.00 | 0.00 | 0.00 | 0.00 | 0.00 | 0.00 | 0.00 | 0.00 | 0.00 | 0.00 | 0.00 | 0.00 | 0.00 | 0.00 | 0.00 | 0.00 | 0.00 | 0.00 | 0.00 | 0.00 | 0.00 | 0.00 | 0.00 | 0.00 | 0.00 | 0.00 | 0.00 | 0.00 | 0.00 | 0.00 | 0.00 | 0.00 | 0.00 | 0.00 | 0.00 | 0.00 | 0.00 | 0.00 | 0.00 | 0.00 | 0.00 | 0.00 | 0.00 | 0.00 | 0.00 | 0.00 | 0.00 | 0.00 | 0.00 | 0.00 | 0.00 | 0.00 | 0.00 | 0.00 | 0.00 | 0.00 | 0.00 | 0.00 | 0.00 | 0.00 | 0.00 | 0.00 | 0.00 | 0.00 | 0.00 | 0.00 | 0.00 | 0.00 | 0.00 | 0.00 | 0.00 | 0.00 | 0.00 | 0.00 | 0.00 | 0.00 | 0.00 | 0.00 | 0.00 | 0.00 | 0.00 | 0.00 | 0.00 | 0.00 | 0.00 | 0.00 | 0.00 | 0.01 | 0.01 | 0.01 | 0.01 | 0.01 | 0.00 | 0.00 | 0.00 | 0.00 | 0.00 | 0.00 | 0.00 |
AU12_r | 0.00 | 0.00 | 0.00 | 0.00 | 0.00 | 0.00 | 0.00 | 0.00 | 0.00 | 0.00 | 0.00 | 0.00 | 0.00 | 0.00 | 0.00 | 0.00 | 0.00 | 0.00 | 0.00 | 0.00 | 0.00 | 0.00 | 0.00 | 0.00 | 0.00 | 0.00 | 0.00 | 0.00 | 0.00 | 0.00 | 0.00 | 0.00 | 0.00 | 0.00 | 0.00 | 0.00 | 0.00 | 0.00 | 0.00 | 0.00 | 0.00 | 0.00 | 0.00 | 0.00 | 0.00 | 0.00 | 0.00 | 0.00 | 0.00 | 0.00 | 0.00 | 0.00 | 0.00 | 0.00 | 0.00 | 0.00 | 0.00 | 0.00 | 0.00 | 0.00 | 0.00 | 0.00 | 0.00 | 0.00 | 0.00 | 0.00 | 0.00 | 0.00 | 0.00 | 0.00 | 0.00 | 0.00 | 0.00 | 0.00 | 0.00 | 0.00 | 0.00 | 0.00 | 0.00 | 0.00 | 0.00 | 0.00 | 0.00 | 0.00 | 0.00 | 0.00 | 0.00 | 0.00 | 0.00 | 0.00 | 0.00 | 0.00 | 0.00 | 0.00 | 0.00 | 0.00 | 0.00 | 0.00 | 0.00 | 0.00 | 0.00 |
AU14_r | 0.00 | 0.00 | 0.00 | 0.00 | 0.00 | 0.00 | 0.00 | 0.00 | 0.00 | 0.00 | 0.00 | 0.00 | 0.00 | 0.00 | 0.00 | 0.00 | 0.00 | 0.00 | 0.00 | 0.00 | 0.00 | 0.00 | 0.00 | 0.00 | 0.00 | 0.00 | 0.00 | 0.00 | 0.00 | 0.00 | 0.00 | 0.00 | 0.00 | 0.00 | 0.00 | 0.00 | 0.00 | 0.00 | 0.00 | 0.00 | 0.00 | 0.00 | 0.00 | 0.00 | 0.00 | 0.00 | 0.00 | 0.00 | 0.00 | 0.00 | 0.00 | 0.00 | 0.00 | 0.00 | 0.00 | 0.00 | 0.00 | 0.00 | 0.00 | 0.00 | 0.00 | 0.00 | 0.00 | 0.00 | 0.00 | 0.00 | 0.00 | 0.00 | 0.00 | 0.00 | 0.00 | 0.00 | 0.00 | 0.00 | 0.00 | 0.00 | 0.00 | 0.00 | 0.00 | 0.00 | 0.00 | 0.00 | 0.00 | 0.00 | 0.00 | 0.00 | 0.00 | 0.00 | 0.01 | 0.01 | 0.01 | 0.00 | 0.00 | 0.00 | 0.02 | 0.02 | 0.02 | 0.05 | 0.05 | 0.04 | 0.02 |
AU17_r | 0.00 | 0.00 | 0.00 | 0.00 | 0.00 | 0.00 | 0.00 | 0.00 | 0.00 | 0.00 | 0.00 | 0.00 | 0.00 | 0.00 | 0.00 | 0.00 | 0.00 | 0.00 | 0.00 | 0.00 | 0.00 | 0.00 | 0.00 | 0.00 | 0.00 | 0.00 | 0.00 | 0.00 | 0.00 | 0.00 | 0.00 | 0.00 | 0.01 | 0.01 | 0.01 | 0.01 | 0.01 | 0.00 | 0.00 | 0.00 | 0.00 | 0.01 | 0.00 | 0.00 | 0.00 | 0.00 | 0.00 | 0.00 | 0.00 | 0.00 | 0.00 | 0.00 | 0.00 | 0.00 | 0.00 | 0.00 | 0.00 | 0.00 | 0.00 | 0.00 | 0.00 | 0.00 | 0.00 | 0.00 | 0.00 | 0.00 | 0.01 | 0.00 | 0.00 | 0.00 | 0.00 | 0.00 | 0.00 | 0.00 | 0.00 | 0.00 | 0.00 | 0.00 | 0.00 | 0.00 | 0.00 | 0.00 | 0.00 | 0.00 | 0.00 | 0.00 | 0.00 | 0.00 | 0.00 | 0.01 | 0.01 | 0.01 | 0.01 | 0.01 | 0.01 | 0.01 | 0.00 | 0.01 | 0.00 | 0.00 | 0.00 |
AU20_r | 0.00 | 0.00 | 0.00 | 0.00 | 0.00 | 0.00 | 0.00 | 0.00 | 0.00 | 0.00 | 0.00 | 0.00 | 0.00 | 0.00 | 0.00 | 0.00 | 0.00 | 0.00 | 0.00 | 0.00 | 0.00 | 0.00 | 0.00 | 0.00 | 0.00 | 0.00 | 0.00 | 0.00 | 0.00 | 0.00 | 0.00 | 0.00 | 0.00 | 0.00 | 0.00 | 0.00 | 0.00 | 0.00 | 0.00 | 0.00 | 0.00 | 0.00 | 0.00 | 0.00 | 0.01 | 0.01 | 0.00 | 0.00 | 0.00 | 0.00 | 0.00 | 0.00 | 0.00 | 0.00 | 0.00 | 0.00 | 0.00 | 0.00 | 0.00 | 0.00 | 0.00 | 0.00 | 0.00 | 0.00 | 0.00 | 0.00 | 0.00 | 0.00 | 0.00 | 0.00 | 0.00 | 0.00 | 0.00 | 0.01 | 0.00 | 0.00 | 0.00 | 0.00 | 0.00 | 0.00 | 0.00 | 0.00 | 0.00 | 0.00 | 0.01 | 0.01 | 0.01 | 0.01 | 0.01 | 0.00 | 0.00 | 0.00 | 0.00 | 0.00 | 0.01 | 0.01 | 0.01 | 0.02 | 0.03 | 0.03 | 0.01 |
AU23_r | 0.00 | 0.00 | 0.00 | 0.00 | 0.00 | 0.00 | 0.00 | 0.00 | 0.00 | 0.00 | 0.00 | 0.00 | 0.00 | 0.00 | 0.00 | 0.00 | 0.00 | 0.00 | 0.00 | 0.00 | 0.00 | 0.00 | 0.00 | 0.00 | 0.00 | 0.00 | 0.00 | 0.00 | 0.00 | 0.00 | 0.00 | 0.00 | 0.00 | 0.00 | 0.00 | 0.00 | 0.00 | 0.00 | 0.00 | 0.00 | 0.00 | 0.00 | 0.00 | 0.00 | 0.00 | 0.00 | 0.00 | 0.00 | 0.00 | 0.00 | 0.00 | 0.00 | 0.00 | 0.00 | 0.00 | 0.00 | 0.00 | 0.00 | 0.00 | 0.00 | 0.00 | 0.00 | 0.00 | 0.00 | 0.00 | 0.00 | 0.00 | 0.00 | 0.00 | 0.00 | 0.00 | 0.00 | 0.00 | 0.00 | 0.00 | 0.00 | 0.00 | 0.00 | 0.00 | 0.00 | 0.00 | 0.00 | 0.00 | 0.00 | 0.00 | 0.00 | 0.00 | 0.00 | 0.00 | 0.00 | 0.00 | 0.00 | 0.00 | 0.00 | 0.00 | 0.00 | 0.00 | 0.00 | 0.00 | 0.00 | 0.00 |
AU25_r | 0.00 | 0.00 | 0.00 | 0.00 | 0.00 | 0.00 | 0.00 | 0.00 | 0.00 | 0.00 | 0.00 | 0.00 | 0.00 | 0.00 | 0.00 | 0.00 | 0.00 | 0.00 | 0.00 | 0.00 | 0.00 | 0.00 | 0.00 | 0.00 | 0.00 | 0.00 | 0.00 | 0.00 | 0.00 | 0.00 | 0.00 | 0.00 | 0.00 | 0.00 | 0.00 | 0.00 | 0.00 | 0.00 | 0.00 | 0.00 | 0.00 | 0.00 | 0.00 | 0.00 | 0.00 | 0.00 | 0.00 | 0.00 | 0.00 | 0.00 | 0.00 | 0.00 | 0.00 | 0.00 | 0.00 | 0.00 | 0.00 | 0.00 | 0.00 | 0.00 | 0.00 | 0.00 | 0.00 | 0.00 | 0.00 | 0.00 | 0.00 | 0.00 | 0.00 | 0.00 | 0.00 | 0.00 | 0.00 | 0.00 | 0.00 | 0.00 | 0.00 | 0.00 | 0.00 | 0.00 | 0.00 | 0.00 | 0.00 | 0.00 | 0.00 | 0.00 | 0.00 | 0.00 | 0.00 | 0.00 | 0.01 | 0.01 | 0.01 | 0.00 | 0.01 | 0.01 | 0.01 | 0.01 | 0.01 | 0.01 | 0.01 |
AU26_r | 0.00 | 0.00 | 0.00 | 0.00 | 0.00 | 0.00 | 0.00 | 0.00 | 0.00 | 0.00 | 0.00 | 0.00 | 0.00 | 0.00 | 0.00 | 0.01 | 0.00 | 0.00 | 0.01 | 0.00 | 0.00 | 0.00 | 0.00 | 0.00 | 0.00 | 0.00 | 0.00 | 0.00 | 0.00 | 0.00 | 0.00 | 0.00 | 0.00 | 0.00 | 0.00 | 0.00 | 0.00 | 0.00 | 0.00 | 0.00 | 0.00 | 0.00 | 0.00 | 0.00 | 0.00 | 0.00 | 0.00 | 0.00 | 0.00 | 0.00 | 0.00 | 0.00 | 0.00 | 0.00 | 0.00 | 0.00 | 0.00 | 0.00 | 0.00 | 0.00 | 0.00 | 0.00 | 0.00 | 0.00 | 0.00 | 0.00 | 0.00 | 0.00 | 0.01 | 0.00 | 0.00 | 0.01 | 0.00 | 0.00 | 0.00 | 0.00 | 0.00 | 0.00 | 0.00 | 0.00 | 0.00 | 0.00 | 0.00 | 0.00 | 0.00 | 0.01 | 0.01 | 0.01 | 0.01 | 0.01 | 0.01 | 0.01 | 0.00 | 0.00 | 0.01 | 0.01 | 0.01 | 0.01 | 0.01 | 0.01 | 0.01 |
AU45_r | 0.00 | 0.00 | 0.00 | 0.00 | 0.00 | 0.00 | 0.00 | 0.00 | 0.00 | 0.00 | 0.00 | 0.00 | 0.00 | 0.00 | 0.00 | 0.00 | 0.00 | 0.00 | 0.00 | 0.00 | 0.00 | 0.00 | 0.00 | 0.00 | 0.00 | 0.00 | 0.00 | 0.00 | 0.00 | 0.00 | 0.00 | 0.00 | 0.00 | 0.00 | 0.01 | 0.01 | 0.01 | 0.02 | 0.02 | 0.02 | 0.02 | 0.02 | 0.01 | 0.00 | 0.00 | 0.00 | 0.01 | 0.01 | 0.01 | 0.01 | 0.01 | 0.00 | 0.00 | 0.00 | 0.00 | 0.00 | 0.01 | 0.00 | 0.00 | 0.00 | 0.01 | 0.01 | 0.01 | 0.02 | 0.02 | 0.02 | 0.01 | 0.01 | 0.00 | 0.00 | 0.00 | 0.00 | 0.00 | 0.00 | 0.00 | 0.00 | 0.00 | 0.00 | 0.00 | 0.00 | 0.00 | 0.00 | 0.00 | 0.00 | 0.00 | 0.00 | 0.00 | 0.00 | 0.00 | 0.00 | 0.00 | 0.00 | 0.00 | 0.00 | 0.00 | 0.00 | 0.00 | 0.00 | 0.00 | 0.00 | 0.00 |
[23]:
cumulative_complexity_peaks_df, significant_peaks_df = cumulative_complexity_peaks(df=complexity_resonance_df)
[24]:
_ = cumulative_complexity_peaks_plot(cumulative_complexity_peaks_df=cumulative_complexity_peaks_df,
significant_peaks_df=significant_peaks_df,
)
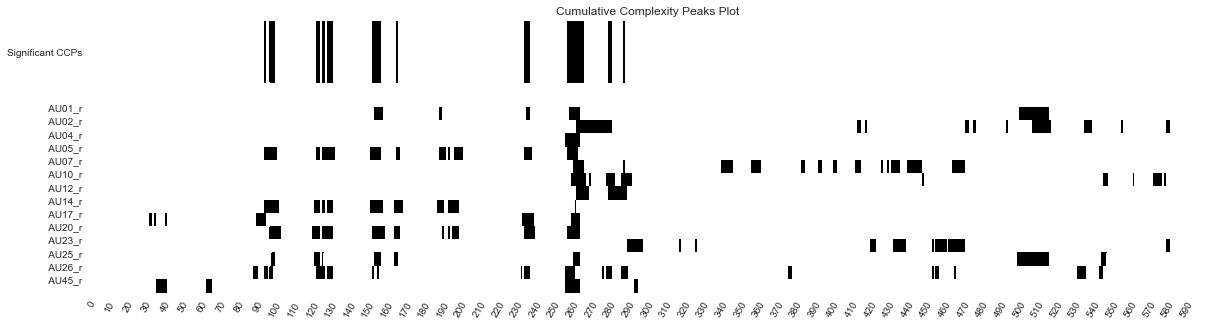
Ts_levels example feature¶
[25]:
example_feature = scaled_ts_df.columns[0]
[26]:
ts_levels_df, fig, ax = ts_levels(ts=scaled_ts_df[example_feature],
ts_x=None,
criterion="mse",
max_depth=10,
min_samples_leaf=15,
min_samples_split=2,
max_leaf_nodes=30,
plot=True,
equal_spaced=True,
n_x_ticks=10)
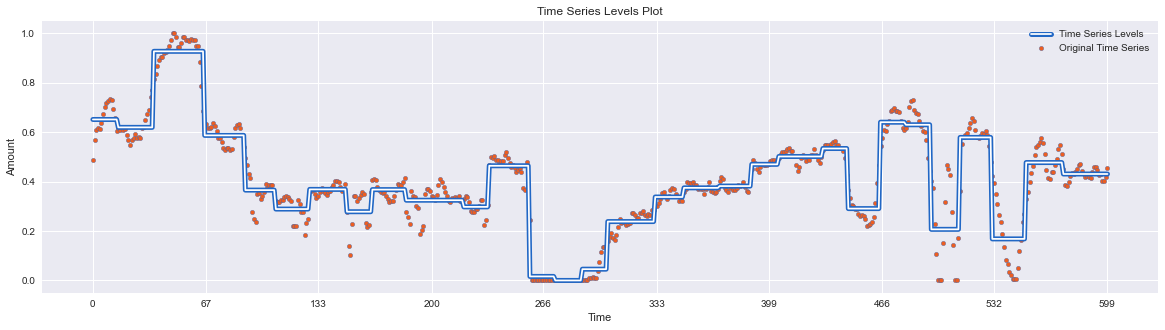
Plot all of the time series levels of the FACS¶
[27]:
all_ts_levels = pd.DataFrame()
for column in scaled_ts_df.columns.tolist():
ts_levels_df, fig, ax = ts_levels(ts=scaled_ts_df[column],
ts_x=None,
criterion="mse",
max_depth=10,
min_samples_leaf=15,
min_samples_split=2,
max_leaf_nodes=30,
plot=False,
equal_spaced=True,
n_x_ticks=10)
_ = ts_levels_df.set_index("t_steps", inplace=True)
ts_levels_df.columns = [f"{column[1:]}_{x}" for x in ts_levels_df.columns.tolist()]
all_ts_levels = pd.concat([all_ts_levels, ts_levels_df], axis=1)
[28]:
plot_df = (all_ts_levels
.reset_index()
.melt(id_vars="t_steps"))
[29]:
plt.figure(figsize=(30, 10))
ax = sns.lineplot(x="t_steps",
y="value",
hue="variable",
palette="gist_rainbow",
data=plot_df[plot_df["variable"].str.contains("original_ts")],
legend=False,
linestyle='dashed',
)
ax = sns.lineplot(x="t_steps",
y="value",
hue="variable",
palette="gist_rainbow",
data=plot_df[plot_df["variable"].str.contains("ts_levels")],
)
_ = ax.set(xlabel='Time steps', ylabel='Facial Action Units',
title='Different facial action units ts-levels')
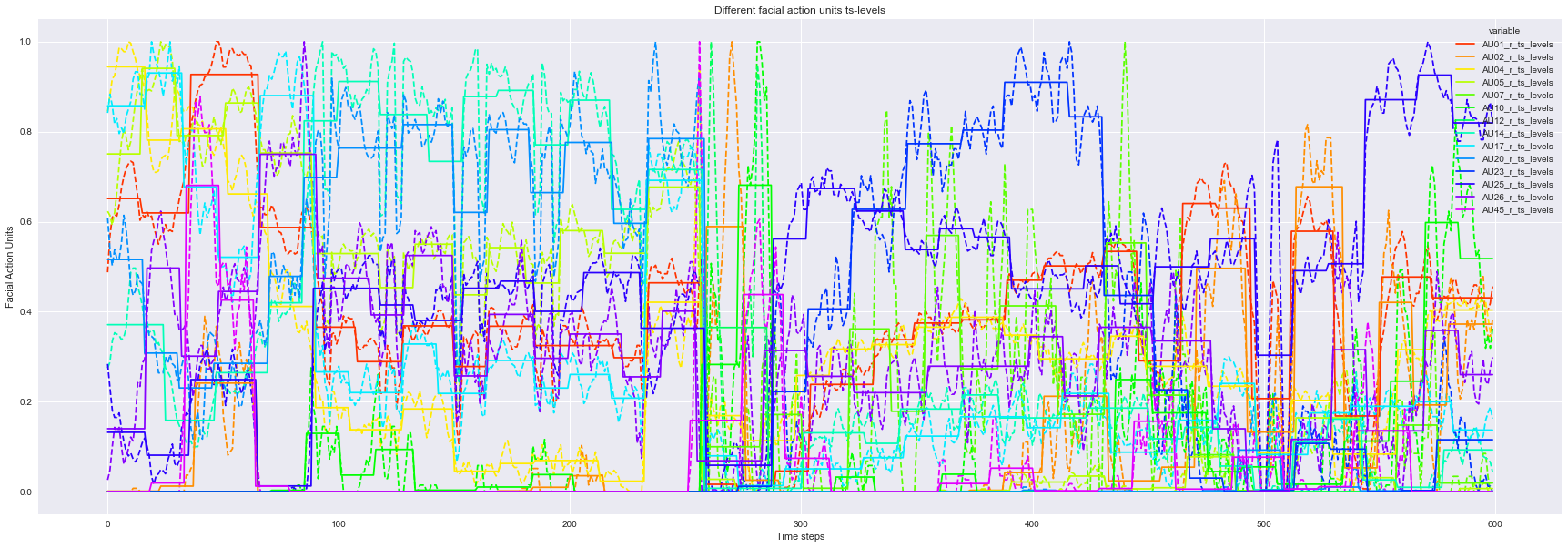