Using a toy dataset to better understand SHapley Additive exPlanations (SHAP)¶
[1]:
import pandas as pd
import math
import numpy as np
import seaborn as sns
import matplotlib.pyplot as plt
import shap
from jmspack.utils import apply_scaling, JmsColors
from jmspack.ml_utils import multi_roc_auc_plot, optimize_model, plot_confusion_matrix, summary_performance_metrics_classification, plot_learning_curve, plot_decision_boundary
shap.initjs()
/opt/miniconda3/envs/ds_env/lib/python3.10/site-packages/tqdm/auto.py:22: TqdmWarning: IProgress not found. Please update jupyter and ipywidgets. See https://ipywidgets.readthedocs.io/en/stable/user_install.html
from .autonotebook import tqdm as notebook_tqdm
[2]:
from sklearn.ensemble import RandomForestClassifier
from sklearn.linear_model import LogisticRegression
from sklearn.model_selection import cross_validate, RepeatedStratifiedKFold
[3]:
if "jms_style_sheet" in plt.style.available:
plt.style.use("jms_style_sheet")
[4]:
df = pd.read_csv("https://raw.githubusercontent.com/mwaskom/seaborn-data/master/penguins.csv").dropna()
[5]:
df.head()
[5]:
species | island | bill_length_mm | bill_depth_mm | flipper_length_mm | body_mass_g | sex | |
---|---|---|---|---|---|---|---|
0 | Adelie | Torgersen | 39.1 | 18.7 | 181.0 | 3750.0 | MALE |
1 | Adelie | Torgersen | 39.5 | 17.4 | 186.0 | 3800.0 | FEMALE |
2 | Adelie | Torgersen | 40.3 | 18.0 | 195.0 | 3250.0 | FEMALE |
4 | Adelie | Torgersen | 36.7 | 19.3 | 193.0 | 3450.0 | FEMALE |
5 | Adelie | Torgersen | 39.3 | 20.6 | 190.0 | 3650.0 | MALE |
[6]:
df.info()
<class 'pandas.core.frame.DataFrame'>
Int64Index: 333 entries, 0 to 343
Data columns (total 7 columns):
# Column Non-Null Count Dtype
--- ------ -------------- -----
0 species 333 non-null object
1 island 333 non-null object
2 bill_length_mm 333 non-null float64
3 bill_depth_mm 333 non-null float64
4 flipper_length_mm 333 non-null float64
5 body_mass_g 333 non-null float64
6 sex 333 non-null object
dtypes: float64(4), object(3)
memory usage: 20.8+ KB
[7]:
_ = sns.pairplot(data=df, hue="species", height=1.5)
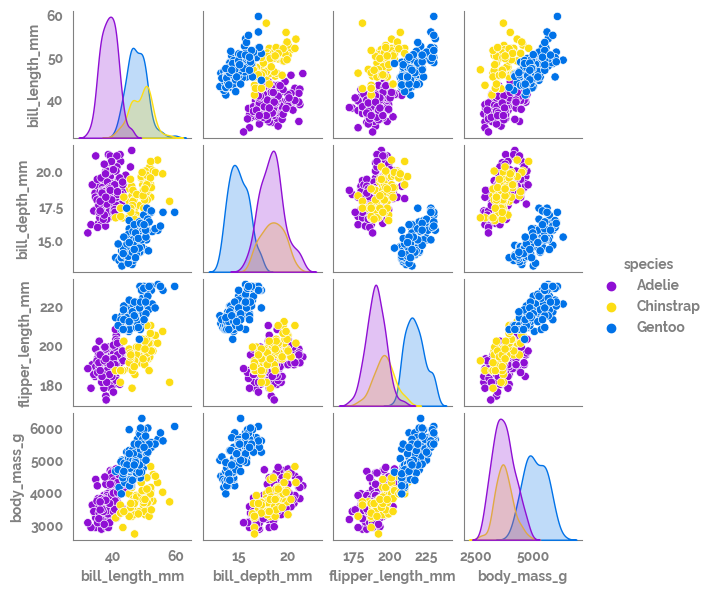
[8]:
_ = sns.pairplot(data=df, hue="sex", height=1.5)
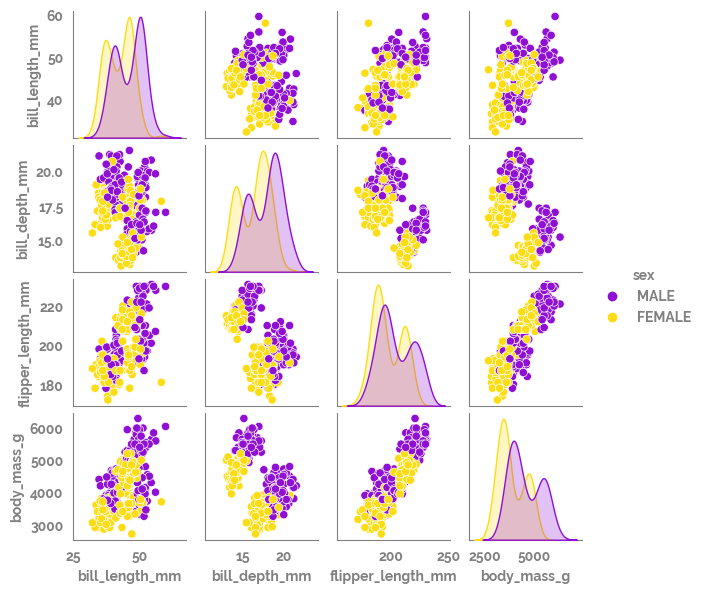
[9]:
features_list = df.select_dtypes("number").columns.tolist()
target = "sex"
X = df[features_list]
y = df[target]
[10]:
(optimized_estimator,
feature_ranking,
feature_selected,
feature_importance,
optimized_params_df,
) = optimize_model(X=X, y=y, estimator= RandomForestClassifier(),
grid_params_dict = {
"max_depth": [1, 2, 3, 4, 5, 10],
"n_estimators": [10, 20, 30, 40, 50],
"max_features": ["log2", "auto", "sqrt"],
"criterion": ["gini", "entropy"],
},
gridsearch_kwargs = {"scoring": "roc_auc", "cv": 3, "n_jobs": -2},
rfe_kwargs = {"n_features_to_select": 2, "verbose": 1})
Fitting estimator with 4 features.
Fitting estimator with 3 features.
- Sizes :
- X shape = (333, 4)
- y shape = (333,)
- X_train shape = (233, 4)
- X_test shape = (100, 4)
- y_train shape = (233,)
- y_test shape = (100,)
- Model info :
- Optimal Parameters = {'bootstrap': True, 'ccp_alpha': 0.0, 'class_weight': None, 'criterion': 'entropy', 'max_depth': 10, 'max_features': 'auto', 'max_leaf_nodes': None, 'max_samples': None, 'min_impurity_decrease': 0.0, 'min_samples_leaf': 1, 'min_samples_split': 2, 'min_weight_fraction_leaf': 0.0, 'n_estimators': 30, 'n_jobs': None, 'oob_score': False, 'random_state': None, 'verbose': 0, 'warm_start': False}
- Selected feature list = ['bill_depth_mm', 'body_mass_g']
- Accuracy score on test set = 84.0%
[11]:
optimized_params_df
[11]:
bootstrap | ccp_alpha | class_weight | criterion | max_depth | max_features | max_leaf_nodes | max_samples | min_impurity_decrease | min_samples_leaf | min_samples_split | min_weight_fraction_leaf | n_estimators | n_jobs | oob_score | random_state | verbose | warm_start | |
---|---|---|---|---|---|---|---|---|---|---|---|---|---|---|---|---|---|---|
optimal_parameters | True | 0.0 | None | entropy | 10 | auto | None | None | 0.0 | 1 | 2 | 0.0 | 30 | None | False | None | 0 | False |
[12]:
feature_importance_df = pd.DataFrame(feature_importance.sort_values(ascending=False)).reset_index().rename(columns={"index": "feature", 0: "feature_importance"})
[13]:
_ = plt.figure(figsize=(7, 3))
_ = sns.barplot(data=feature_importance_df, x="feature_importance", y="feature")
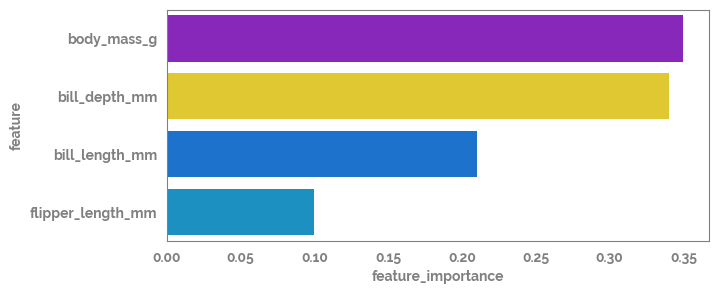
[14]:
explainer = shap.TreeExplainer(optimized_estimator)
shap_values = explainer(X)
[15]:
shap.plots.beeswarm(shap_values[:, :, 1])
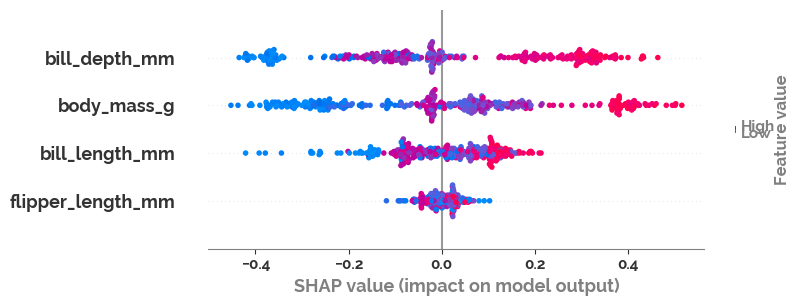
[16]:
shap_actual_df = pd.concat([pd.DataFrame(shap_values[:, :, 1].values, columns=features_list).melt(value_name="shap_data"),
pd.DataFrame(shap_values[:, :, 1].data, columns=features_list).melt(value_name="actual_data").drop("variable", axis=1)], axis=1)
[17]:
shap_actual_df.info()
<class 'pandas.core.frame.DataFrame'>
RangeIndex: 1332 entries, 0 to 1331
Data columns (total 3 columns):
# Column Non-Null Count Dtype
--- ------ -------------- -----
0 variable 1332 non-null object
1 shap_data 1332 non-null float64
2 actual_data 1332 non-null float64
dtypes: float64(2), object(1)
memory usage: 31.3+ KB
[18]:
shap_actual_df
[18]:
variable | shap_data | actual_data | |
---|---|---|---|
0 | bill_length_mm | 0.086101 | 39.1 |
1 | bill_length_mm | -0.023608 | 39.5 |
2 | bill_length_mm | 0.032321 | 40.3 |
3 | bill_length_mm | -0.280766 | 36.7 |
4 | bill_length_mm | 0.021243 | 39.3 |
... | ... | ... | ... |
1327 | body_mass_g | -0.003304 | 4925.0 |
1328 | body_mass_g | -0.032694 | 4850.0 |
1329 | body_mass_g | 0.376044 | 5750.0 |
1330 | body_mass_g | 0.257553 | 5200.0 |
1331 | body_mass_g | 0.408728 | 5400.0 |
1332 rows × 3 columns
[19]:
import matplotlib
from mpl_toolkits.axes_grid1.inset_locator import inset_axes
ax = sns.stripplot(data=shap_actual_df,
x="shap_data",
y="variable",
hue="actual_data",
order=shap_actual_df.groupby("variable").var()["shap_data"].sort_values(ascending=False).index.tolist(),
alpha=0.5,
palette="viridis")
_ = plt.legend([],[], frameon=False)
N = 2
cmap = matplotlib.cm.get_cmap('viridis')
cmap_values = np.linspace(0., 1., N)
colors = cmap(cmap_values)
norm = matplotlib.colors.Normalize(vmin=shap_actual_df["actual_data"].min(), vmax=shap_actual_df["actual_data"].max())
# vertical colorbar
cbaxes = inset_axes(plt.gca(), width="3%", height="80%", loc="lower right")
cbar = matplotlib.colorbar.ColorbarBase(cbaxes, cmap=cmap,
norm=norm,
# ticks=ticks
)
cbar.set_label('scale')
# cbar.ax.set_yticklabels(ticks, fontsize=12)
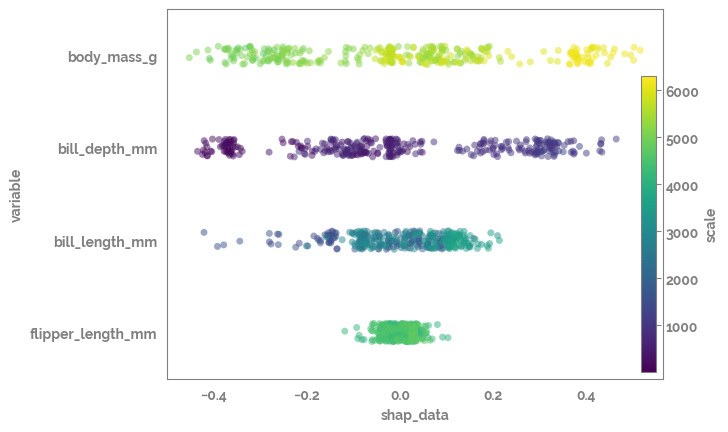
[20]:
shap.summary_plot(shap_values[:, :, 1], X, plot_type="layered_violin", color='coolwarm')
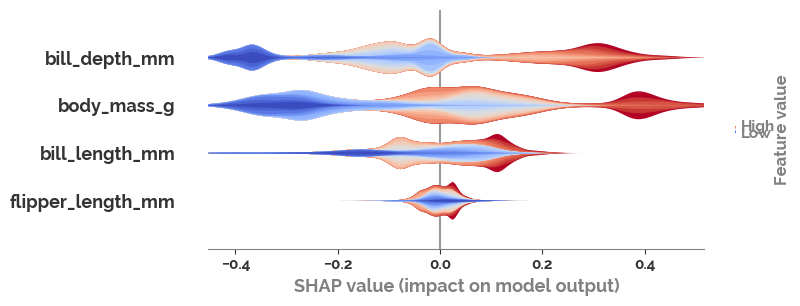
[21]:
shap.plots.heatmap(shap_values[:, :, 1], instance_order=shap_values[:, :, 1].sum(1))
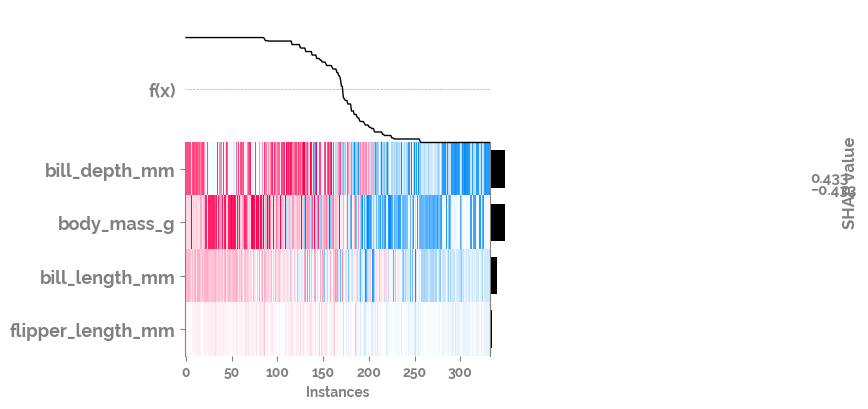
[22]:
# shap.dependence_plot('bill_length_mm', shap_values.values[:, :, 0], X)
shap.force_plot(explainer.expected_value[0],
shap_values.values[:, :, 0]
)
[22]:
Visualization omitted, Javascript library not loaded!
Have you run `initjs()` in this notebook? If this notebook was from another user you must also trust this notebook (File -> Trust notebook). If you are viewing this notebook on github the Javascript has been stripped for security. If you are using JupyterLab this error is because a JupyterLab extension has not yet been written.
Have you run `initjs()` in this notebook? If this notebook was from another user you must also trust this notebook (File -> Trust notebook). If you are viewing this notebook on github the Javascript has been stripped for security. If you are using JupyterLab this error is because a JupyterLab extension has not yet been written.
[23]:
shap.dependence_plot('bill_length_mm', shap_values.values[:, :, 0], X)
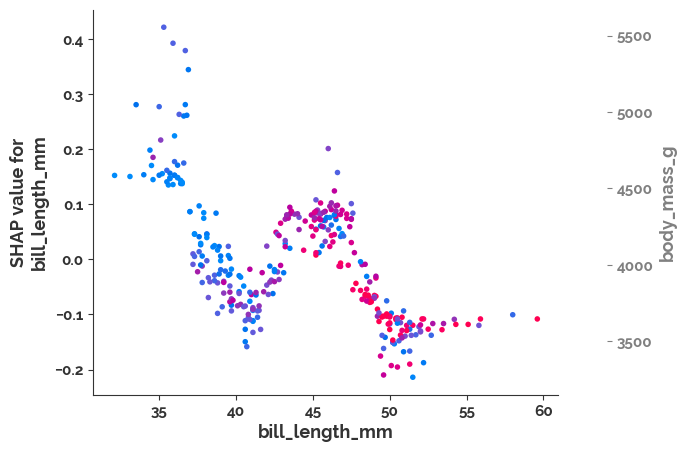
[24]:
# shap.plots.scatter(shap_values.values["bill_length_mm"], color=shap_values.values[:, :, 1])
# shap.plots.scatter(shap_values[:,"Age"])
[25]:
shap_values.values[:, :, 0]
[25]:
array([[-0.08610135, -0.27917051, -0.05639864, -0.07031806],
[ 0.02360813, 0.22710734, 0.08169787, 0.07559811],
[-0.03232096, 0.10554203, 0.01090091, 0.39055613],
...,
[-0.10664765, 0.02194532, -0.0312427 , -0.37604353],
[ 0.05380299, 0.33913148, 0.00596348, -0.25755317],
[-0.11747246, 0.02249328, 0.0117189 , -0.40872828]])
[26]:
shap_values[:, :, 1]
[26]:
.values =
array([[ 0.08610135, 0.27917051, 0.05639864, 0.07031806],
[-0.02360813, -0.22710734, -0.08169787, -0.07559811],
[ 0.03232096, -0.10554203, -0.01090091, -0.39055613],
...,
[ 0.10664765, -0.02194532, 0.0312427 , 0.37604353],
[-0.05380299, -0.33913148, -0.00596348, 0.25755317],
[ 0.11747246, -0.02249328, -0.0117189 , 0.40872828]])
.base_values =
array([0.50801144, 0.50801144, 0.50801144, 0.50801144, 0.50801144,
0.50801144, 0.50801144, 0.50801144, 0.50801144, 0.50801144,
0.50801144, 0.50801144, 0.50801144, 0.50801144, 0.50801144,
0.50801144, 0.50801144, 0.50801144, 0.50801144, 0.50801144,
0.50801144, 0.50801144, 0.50801144, 0.50801144, 0.50801144,
0.50801144, 0.50801144, 0.50801144, 0.50801144, 0.50801144,
0.50801144, 0.50801144, 0.50801144, 0.50801144, 0.50801144,
0.50801144, 0.50801144, 0.50801144, 0.50801144, 0.50801144,
0.50801144, 0.50801144, 0.50801144, 0.50801144, 0.50801144,
0.50801144, 0.50801144, 0.50801144, 0.50801144, 0.50801144,
0.50801144, 0.50801144, 0.50801144, 0.50801144, 0.50801144,
0.50801144, 0.50801144, 0.50801144, 0.50801144, 0.50801144,
0.50801144, 0.50801144, 0.50801144, 0.50801144, 0.50801144,
0.50801144, 0.50801144, 0.50801144, 0.50801144, 0.50801144,
0.50801144, 0.50801144, 0.50801144, 0.50801144, 0.50801144,
0.50801144, 0.50801144, 0.50801144, 0.50801144, 0.50801144,
0.50801144, 0.50801144, 0.50801144, 0.50801144, 0.50801144,
0.50801144, 0.50801144, 0.50801144, 0.50801144, 0.50801144,
0.50801144, 0.50801144, 0.50801144, 0.50801144, 0.50801144,
0.50801144, 0.50801144, 0.50801144, 0.50801144, 0.50801144,
0.50801144, 0.50801144, 0.50801144, 0.50801144, 0.50801144,
0.50801144, 0.50801144, 0.50801144, 0.50801144, 0.50801144,
0.50801144, 0.50801144, 0.50801144, 0.50801144, 0.50801144,
0.50801144, 0.50801144, 0.50801144, 0.50801144, 0.50801144,
0.50801144, 0.50801144, 0.50801144, 0.50801144, 0.50801144,
0.50801144, 0.50801144, 0.50801144, 0.50801144, 0.50801144,
0.50801144, 0.50801144, 0.50801144, 0.50801144, 0.50801144,
0.50801144, 0.50801144, 0.50801144, 0.50801144, 0.50801144,
0.50801144, 0.50801144, 0.50801144, 0.50801144, 0.50801144,
0.50801144, 0.50801144, 0.50801144, 0.50801144, 0.50801144,
0.50801144, 0.50801144, 0.50801144, 0.50801144, 0.50801144,
0.50801144, 0.50801144, 0.50801144, 0.50801144, 0.50801144,
0.50801144, 0.50801144, 0.50801144, 0.50801144, 0.50801144,
0.50801144, 0.50801144, 0.50801144, 0.50801144, 0.50801144,
0.50801144, 0.50801144, 0.50801144, 0.50801144, 0.50801144,
0.50801144, 0.50801144, 0.50801144, 0.50801144, 0.50801144,
0.50801144, 0.50801144, 0.50801144, 0.50801144, 0.50801144,
0.50801144, 0.50801144, 0.50801144, 0.50801144, 0.50801144,
0.50801144, 0.50801144, 0.50801144, 0.50801144, 0.50801144,
0.50801144, 0.50801144, 0.50801144, 0.50801144, 0.50801144,
0.50801144, 0.50801144, 0.50801144, 0.50801144, 0.50801144,
0.50801144, 0.50801144, 0.50801144, 0.50801144, 0.50801144,
0.50801144, 0.50801144, 0.50801144, 0.50801144, 0.50801144,
0.50801144, 0.50801144, 0.50801144, 0.50801144, 0.50801144,
0.50801144, 0.50801144, 0.50801144, 0.50801144, 0.50801144,
0.50801144, 0.50801144, 0.50801144, 0.50801144, 0.50801144,
0.50801144, 0.50801144, 0.50801144, 0.50801144, 0.50801144,
0.50801144, 0.50801144, 0.50801144, 0.50801144, 0.50801144,
0.50801144, 0.50801144, 0.50801144, 0.50801144, 0.50801144,
0.50801144, 0.50801144, 0.50801144, 0.50801144, 0.50801144,
0.50801144, 0.50801144, 0.50801144, 0.50801144, 0.50801144,
0.50801144, 0.50801144, 0.50801144, 0.50801144, 0.50801144,
0.50801144, 0.50801144, 0.50801144, 0.50801144, 0.50801144,
0.50801144, 0.50801144, 0.50801144, 0.50801144, 0.50801144,
0.50801144, 0.50801144, 0.50801144, 0.50801144, 0.50801144,
0.50801144, 0.50801144, 0.50801144, 0.50801144, 0.50801144,
0.50801144, 0.50801144, 0.50801144, 0.50801144, 0.50801144,
0.50801144, 0.50801144, 0.50801144, 0.50801144, 0.50801144,
0.50801144, 0.50801144, 0.50801144, 0.50801144, 0.50801144,
0.50801144, 0.50801144, 0.50801144, 0.50801144, 0.50801144,
0.50801144, 0.50801144, 0.50801144, 0.50801144, 0.50801144,
0.50801144, 0.50801144, 0.50801144, 0.50801144, 0.50801144,
0.50801144, 0.50801144, 0.50801144, 0.50801144, 0.50801144,
0.50801144, 0.50801144, 0.50801144, 0.50801144, 0.50801144,
0.50801144, 0.50801144, 0.50801144, 0.50801144, 0.50801144,
0.50801144, 0.50801144, 0.50801144, 0.50801144, 0.50801144,
0.50801144, 0.50801144, 0.50801144])
.data =
array([[ 39.1, 18.7, 181. , 3750. ],
[ 39.5, 17.4, 186. , 3800. ],
[ 40.3, 18. , 195. , 3250. ],
...,
[ 50.4, 15.7, 222. , 5750. ],
[ 45.2, 14.8, 212. , 5200. ],
[ 49.9, 16.1, 213. , 5400. ]])
[27]:
cv = RepeatedStratifiedKFold(n_splits=10, n_repeats=10, random_state=1)
scoring_list = ('accuracy',
'balanced_accuracy',
'f1',
'f1_weighted',
'precision',
'precision_weighted',
'recall',
'recall_weighted',
'roc_auc',
)
[28]:
tmp_out = cross_validate(estimator=optimized_estimator, X=X[feature_selected], y=y.astype("category").cat.codes, groups=None, scoring=scoring_list, cv=cv, n_jobs=-1)
[29]:
pd.DataFrame(tmp_out).drop(["fit_time", "score_time"], axis=1).agg(["mean", "median", "std"]).round(3)
[29]:
test_accuracy | test_balanced_accuracy | test_f1 | test_f1_weighted | test_precision | test_precision_weighted | test_recall | test_recall_weighted | test_roc_auc | |
---|---|---|---|---|---|---|---|---|---|
mean | 0.871 | 0.871 | 0.872 | 0.870 | 0.872 | 0.876 | 0.878 | 0.871 | 0.942 |
median | 0.879 | 0.879 | 0.882 | 0.879 | 0.882 | 0.884 | 0.882 | 0.879 | 0.949 |
std | 0.058 | 0.058 | 0.058 | 0.058 | 0.072 | 0.057 | 0.080 | 0.058 | 0.043 |
[30]:
cv_metrics_df = pd.DataFrame(tmp_out).drop(["fit_time", "score_time"], axis=1).melt(var_name="Metric")
[31]:
_ = plt.figure(figsize=(25,5))
_ = sns.boxplot(data = cv_metrics_df,
x = "Metric",
y = "value")
_ = sns.swarmplot(data = cv_metrics_df,
x = "Metric",
y = "value", edgecolor="white", linewidth=1)
_ = plt.title(f"All performance metrics {optimized_estimator.__class__.__name__} with cross validation")
7.0% of the points cannot be placed; you may want to decrease the size of the markers or use stripplot.
15.0% of the points cannot be placed; you may want to decrease the size of the markers or use stripplot.
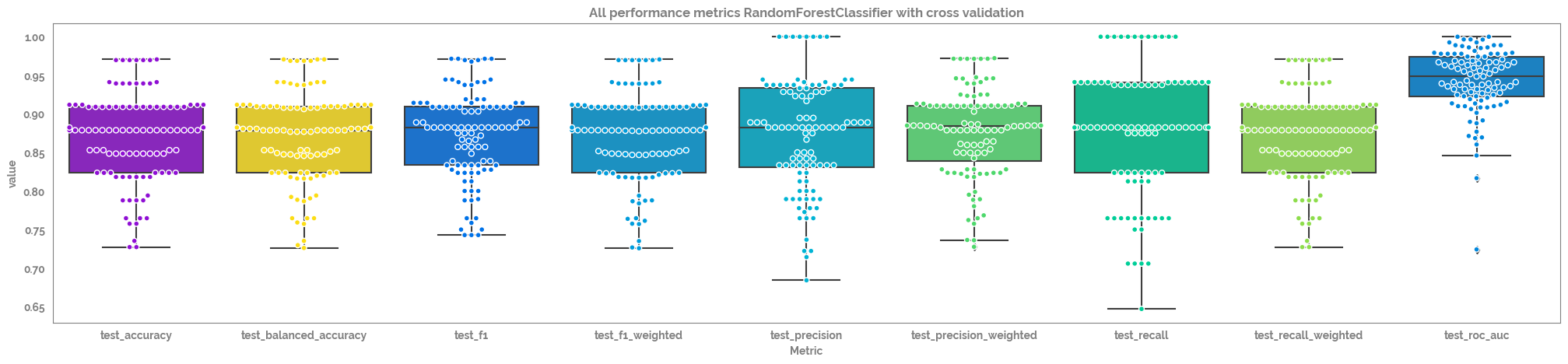
[32]:
fig = plot_learning_curve(estimator=optimized_estimator,
title=f'Learning Curve of {optimized_estimator.__class__.__name__} of penguins',
X=X[feature_selected],
y=y,
groups=None,
cross_color=JmsColors.PURPLE,
test_color=JmsColors.YELLOW,
scoring='roc_auc',
ylim=None,
cv=cv,
n_jobs=10,
train_sizes=np.linspace(.1, 1.0, 40),
figsize=(10,5))
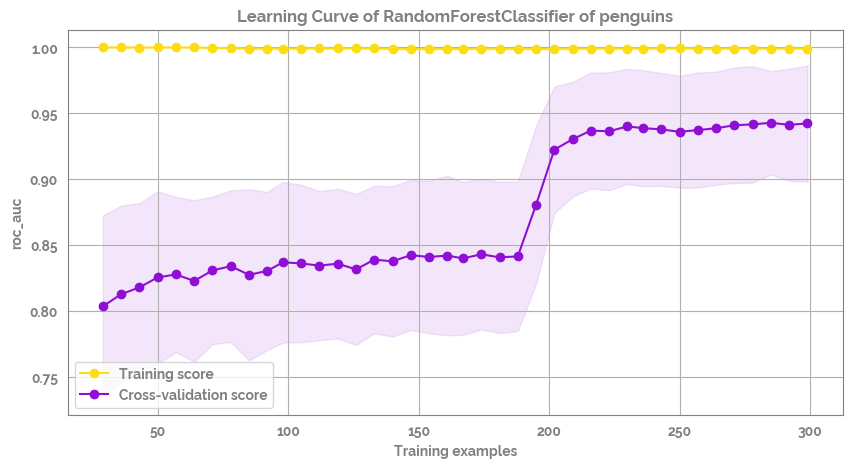
[33]:
_ = plot_decision_boundary(X=X[feature_selected],
y=y,
clf=optimized_estimator,
title = f'Decision Boundary - {optimized_estimator.__class__.__name__}',
legend_title = target,
h=0.1,
figsize=(8, 6))
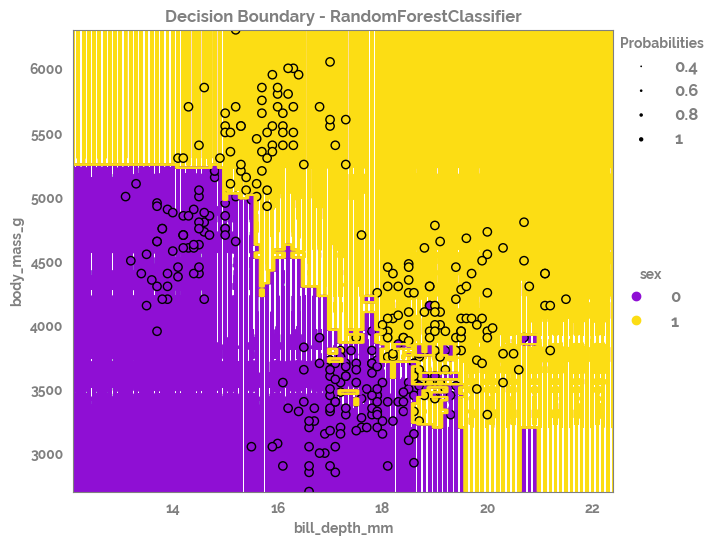
[ ]: